Ở bài học trước, Tui đã trình bày Tổng quan về tích hợp Mô hình Máy học vào hệ thống Kinh doanh – Quản lý, các bạn đã biết được chu trình cần thiết cho việc xây dựng một mô hình máy học rồi. Trong bài này chúng ta tiến hành coding, Tui sẽ dùng Hồi quy tuyến tính để tạo mô hình máy học dự báo giá nhà từ tập dữ liệu mẫu Kaggle (tải ở đây) được lưu dưới định dạng CSV, khoảng 5,000 dòng dữ liệu.
Lý thuyết về hồi quy tuyến tính được Anh Vũ Hữu Tiệp và Cộng Sự trình bày rất kỹ trên blog Machine Learning Cơ Bản nên các bạn đọc trực tiếp trên này, hoặc đọc từ Wikipedia, do đó Tui không có nhắc lại phần lý thuyết.
Part 1 này Tui đi trực tiếp vào ứng dụng giải thuật máy học được cung cấp từ một số thư viện trong Python để tạo mô hình máy học dự báo giá nhà cho tập dữ liệu Kaggle ở trên, sử dụng Pycharm (các bạn có thể dùng Jupyter bằng cách cài đặt Anaconda3) chạy dạng Console, Part 2 Tui sẽ hướng dẫn các bạn cách chạy trên giao diện tương tác người dùng.
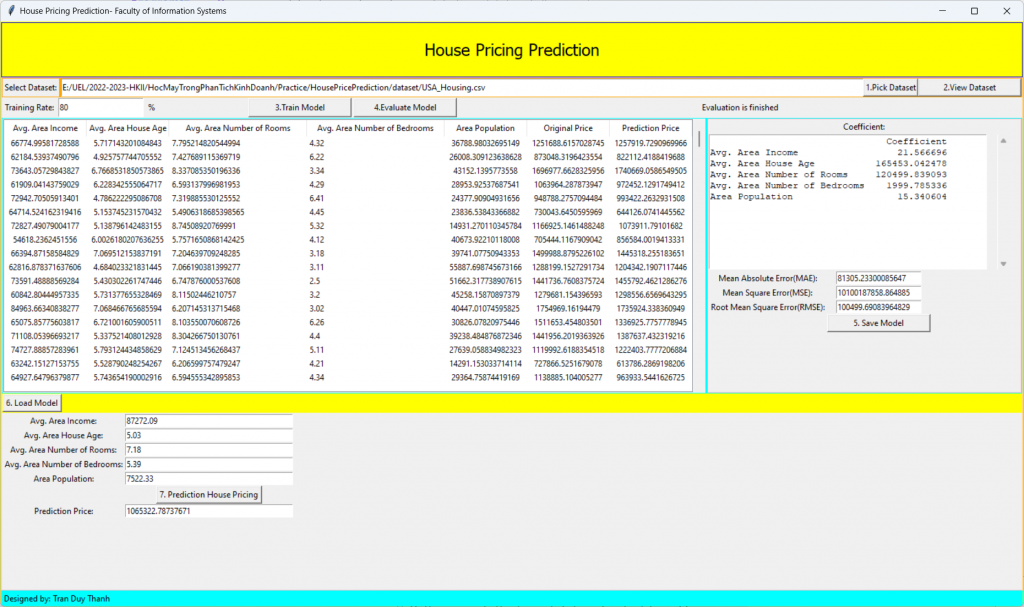
Các bạn thực hiện theo các bước sau:
Bước 1: Tạo dự án tên “HousingPricePrediction” trong Pycharm
Trong Project tạo một thư mục dataset và tải dữ liệu rồi lưu vào thư mục này
https://tranduythanh.com/datasets/USA_Housing.csv
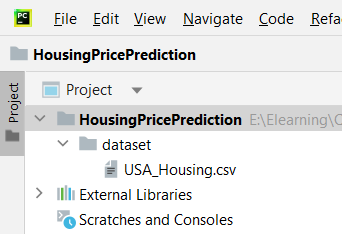
Tui mô tả sơ lược dataset này:
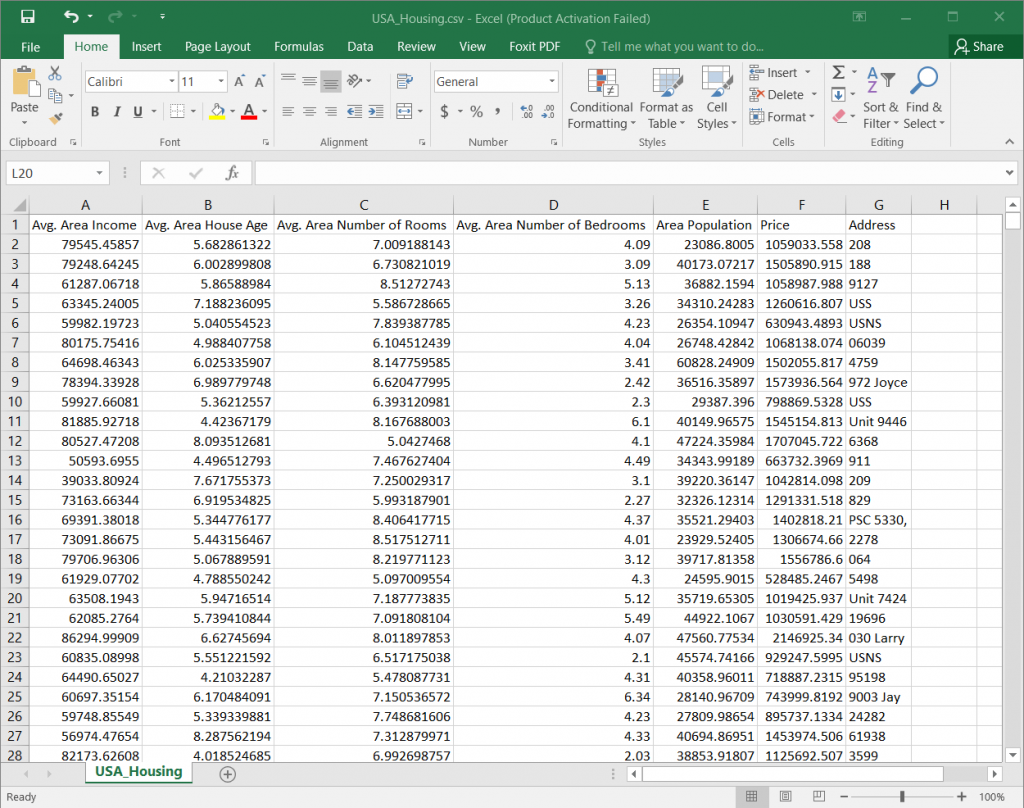
- Avg. Area Income: Thu nhập trung bình tại khu vực ngôi nhà đã bán
- Avg. Area House Age: Trung bình tuổi của một ngôi nhà đã bán (số năm sử dụng)
- Avg. Area Number of Rooms: Trung bình diện tích các phòng
- Avg. Area Number of Bedrooms: Trung bình diện tích phòng ngủ
- Area Population: Dân số tại khu vực bán nhà
- Price: Giá ngôi nhà đã bán
- Address: Địa chỉ ngôi nhà bán
Trong bài này ta không sử dụng thuộc tính Address.
Nhiệm vụ của chúng ta là xây dựng 1 mô hình máy học dựa trên tập dữ liệu này, khi người dùng nhập vào Area Income, House Age, Number of Rooms, Number of Bedroooms, Area Population thì mô hình sẽ dự báo Giá của căn nhà này là bao nhiêu.
Bước 2: Tạo một file Python “HousingPriceSimpleModel.py”:
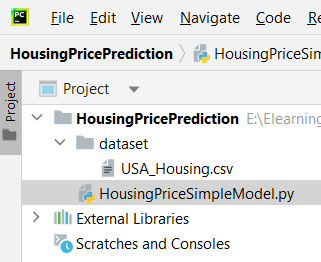
Bước 3: Bổ sung các thư viện ở đầu file “HousingPriceSimpleModel.py”
# 01.Library for dataset processing
import pandas as pd
import numpy as np
# 02. Library for train model
from sklearn.model_selection import train_test_split
from sklearn.linear_model import LinearRegression
# 03. Library for evaluation model
from sklearn import metrics
# 04. Library for saving model
import pickle
Ở trên Tui khai báo 4 nhóm thư viện bao gồm:
- Nhóm thư viện xử lý dataset (01) : Đọc dữ liệu, tiền xử lý loại bỏ cột dữ liệu không sử dụng, trích lọc các biến độc lập (các cột thuộc tính), biến phụ thuộc (cột price)… Chúng ta dùng pandas, numpy
- Nhóm thư viện để train model máy học (02): Bao gồm thư viện tách lọc tập train và test, và bài này chúng ta dùng hồi quy tuyến tính (LinearRegression)
- Nhóm thư viện đánh giá chất lượng mô hình (03): chúng ta dùng thư viện metrics
- Nhóm thư viện để xuất mô hình máy học được train xuống ổ cứng (04): Ta dùng thư viện pickle
Bước 4: Tiếp tục bổ sung mã lệnh đọc dữ liệu, xem các thông tin cũng như tạo ma trận X và mảng y
#use pandas to read CSV dataset
df = pd.read_csv('dataset/USA_Housing.csv')
#call functions about get dataset information:
print(df.head())
print(df.info())
print(df.describe())
print(df.columns)
Thực hiện các Lệnh ở trên ta có kết quả:
Avg. Area Income ... Address
0 79545.458574 ... 208 Michael Ferry Apt. 674\nLaurabury, NE 3701...
1 79248.642455 ... 188 Johnson Views Suite 079\nLake Kathleen, CA...
2 61287.067179 ... 9127 Elizabeth Stravenue\nDanieltown, WI 06482...
3 63345.240046 ... USS Barnett\nFPO AP 44820
4 59982.197226 ... USNS Raymond\nFPO AE 09386
[5 rows x 7 columns]
<class 'pandas.core.frame.DataFrame'>
RangeIndex: 5000 entries, 0 to 4999
Data columns (total 7 columns):
# Column Non-Null Count Dtype
--- ------ -------------- -----
0 Avg. Area Income 5000 non-null float64
1 Avg. Area House Age 5000 non-null float64
2 Avg. Area Number of Rooms 5000 non-null float64
3 Avg. Area Number of Bedrooms 5000 non-null float64
4 Area Population 5000 non-null float64
5 Price 5000 non-null float64
6 Address 5000 non-null object
dtypes: float64(6), object(1)
memory usage: 273.6+ KB
None
Avg. Area Income Avg. Area House Age ... Area Population Price
count 5000.000000 5000.000000 ... 5000.000000 5.000000e+03
mean 68583.108984 5.977222 ... 36163.516039 1.232073e+06
std 10657.991214 0.991456 ... 9925.650114 3.531176e+05
min 17796.631190 2.644304 ... 172.610686 1.593866e+04
25% 61480.562388 5.322283 ... 29403.928702 9.975771e+05
50% 68804.286404 5.970429 ... 36199.406689 1.232669e+06
75% 75783.338666 6.650808 ... 42861.290769 1.471210e+06
max 107701.748378 9.519088 ... 69621.713378 2.469066e+06
[8 rows x 6 columns]
Index(['Avg. Area Income', 'Avg. Area House Age', 'Avg. Area Number of Rooms',
'Avg. Area Number of Bedrooms', 'Area Population', 'Price', 'Address'],
dtype='object')
Process finished with exit code 0
Bước 5: Xây dựng và train mô hình dự báo giá nhà
Ta cần tách dữ liệu thành một ma trận X chứa các đặc trưng cần đào tạo (gọi là các biến độc lập) và một mảng y với biến mục tiêu (biến phụ thuộc), trong trường hợp này thì cột y chính là cột Giá(Price). Cột Địa chỉ (Address) sẽ được loại bỏ khỏi mô hình vì nó chỉ có thông tin văn bản mà mô hình hồi quy tuyến tính này không sử dụng. Điều đó có nghĩa:
#set X matrix
#df.columns[:5] meaning:
#['Avg. Area Income', 'Avg. Area House Age', 'Avg. Area Number of Rooms',
# 'Avg. Area Number of Bedrooms', 'Area Population']
X = df[df.columns[:5]]
y = df['Price']
# Printing for observation:
print(X)
print(y)
Thực hiện các Lệnh ở trên ta có kết quả:
Avg. Area Income ... Area Population
0 79545.458574 ... 23086.800503
1 79248.642455 ... 40173.072174
2 61287.067179 ... 36882.159400
3 63345.240046 ... 34310.242831
4 59982.197226 ... 26354.109472
... ... ... ...
4995 60567.944140 ... 22837.361035
4996 78491.275435 ... 25616.115489
4997 63390.686886 ... 33266.145490
4998 68001.331235 ... 42625.620156
4999 65510.581804 ... 46501.283803
[5000 rows x 5 columns]
0 1.059034e+06
1 1.505891e+06
2 1.058988e+06
3 1.260617e+06
4 6.309435e+05
...
4995 1.060194e+06
4996 1.482618e+06
4997 1.030730e+06
4998 1.198657e+06
4999 1.298950e+06
Name: Price, Length: 5000, dtype: float64
Thông thường ta cần chia tập dữ liệu ra làm 2: train và set. Tỉ lệ tùy thuộc vào dữ liệu cũng như kinh nghiệm. Giả sử ở đây ta chi theo tỉ lệ 80% cho train và 20% cho test.
X_train, X_test, y_train, y_test = train_test_split(X, y, test_size=0.2, random_state=101)
lm = LinearRegression()
lm.fit(X_train, y_train)
Mã lệnh ở trên sẽ tách tập dataset ban đầu thành 2 tập, tập train và tập test với tỉ lệ lần lượt là 80% và 20%, sau đó dùng LinearRegression() để train mô hình máy học.
Bước 6: Thử nghiệm mô hình
Chúng ta có thể đánh giá mô hình sau đó thử nghiệm, ở bước này Tui sẽ thử nghiệm mô hình sau đó sẽ đánh giá lại để quyết định xem có nên xuất ra ổ cứng để sử dụng sau này hay không.
- Thử nghiệm lần thứ 1: Lấy ngẫu nhiên tập dữ liệu thứ 0 trong iloc của X_test
print("Input 1:")
print([X_test.iloc[0]])
pre1 = lm.predict([X_test.iloc[0]])
print("Housing Price prediction 1 =", pre1)
Thực thi lệnh ở trên ta có kết quả:
Input 1:
[Avg. Area Income 66774.995817
Avg. Area House Age 5.717143
Avg. Area Number of Rooms 7.795215
Avg. Area Number of Bedrooms 4.320000
Area Population 36788.980327
Name: 1718, dtype: float64]
Housing Price prediction 1 = [1257919.729097]
So sánh với dữ liệu gốc, với các thông số input ở trên thì ta có dữ liệu giá gốc là (bạn tra lại dữ liệu gốc trong file CSV):
1251688.616
Còn mô hình ta tính ra được:
1257919.729097
Như vậy giá dự báo cũng khá sát với giá gốc
- Thử nghiệm lần thứ 2, các giá trị đầu vào Tui sẽ lấy y chóc như lần thử nghiệm thứ 1, tuy nhiên Tui không dùng iloc[0] của X_test, mà Tui sẽ nhập trực tiếp:
print("Input 2:")
input2=[66774.995817, 5.717143, 7.795215, 4.320000, 36788.980327]
pre2 = lm.predict([input2])
print("Housing Price prediction 2 =", pre2)
Thực thi lệnh ở trên ta có kết quả:
Input 2:
[66774.995817, 5.717143, 7.795215, 4.32, 36788.980327]
Housing Price prediction 2 = [1257919.71744578]
So sánh kết quả so với lần thử nghiêm thứ 1 ta thấy kết quả hầu như chính xác như nhau.
- Thử nghiệm lần thứ 3, Tui sử dụng một mảng dữ liệu đầu vào khác:
print("Input 3:")
input3=[21.566696, 165453.0425, 120499.8391, 1999.785336, 15.340604]
print(input3)
pre3 = lm.predict([input3])
print("Housing Price prediction 3 =", pre3)
Thực thi lệnh ở trên ta có kết quả:
Input 3:
[21.566696, 165453.0425, 120499.8391, 1999.785336, 15.340604]
Housing Price prediction 3 = [4.18962799e+10]
Nếu bạn muốn kiểm tra toàn bộ dữ liệu prediction trên tập test thì có thể code như sau:
predictions = lm.predict(X_test)
print("Full Housing Price Predictions:")
print(predictions)
Thực thi lệnh ở trên ta có kết quả:
Full Housing Price Predictions:
[1257919.729097 822112.41884197 1740669.05865495 972452.12917494
993422.26329315 644126.07414456 1073911.79101682 856584.00194133
1445318.25518365 1204342.19071174 1455792.46212863 1298556.65696433
1735924.33836095 1336925.77577789 1387637.43231922 1222403.77772069
613786.28691982 963933.54416267 1221197.33050139 1198071.57563599
505861.89531673 1769106.54723353 1853881.16842511 1200369.50507868
1065129.1285072 1812033.73067312 1768686.47091262 1439920.83814323
1387251.99649376 1541178.39224181 726418.80504278 1754497.60908688
1462185.72653198 1025600.16081145 1284926.8685155 917454.59590853
1187046.94956865 999330.91117487 1329536.63409595 782191.60441437
1393272.03053841 578216.88394851 822643.37151048 1895533.11413226
1672019.84892041 966926.45434583 1129674.55638146 792797.75914663
1161057.18412143 1472396.71417164 1457656.70412313 1162939.334229
1099453.68110992 1358107.44619413 841103.70380593 986322.30573616
1123323.53002134 1253538.63235181 1428279.66304074 499103.20906177
1462817.08350573 1108744.36856804 660588.51700889 1247031.59801316
1342744.55515752 1357505.99080656 818858.41205464 1487168.7491193
1396247.6559861 885078.37963981 857545.83060842 1212816.45100842
1101069.27148301 1834591.82895367 924022.96601965 855346.8084446
822070.44924512 1397829.2123058 775933.8643152 1638075.3740563
1079223.32119183 1355542.10150295 1321447.90227179 1198773.49593884
757960.00844907 649708.59768215 1058567.42526768 1384318.81434923
1030349.32788272 1297790.45656826 550588.21509211 1706268.87594955
840478.99085965 1627285.73221856 1051446.08561746 947163.26591115
1187965.14618703 1556566.4890801 900538.67981881 995246.18312522
1221693.84495786 1592557.49052096 977671.97252197 1428171.2008612
1015834.61615161 1448110.17850637 877865.27098758 831194.85890213
1022735.97232827 1844169.94971864 1925418.90852406 1405526.2235022
1042730.58532361 1056912.41311355 1440167.01805755 1029466.31854915
1188198.60368704 1407875.62844789 1266581.80632292 1562660.40296843
767006.37537442 1394809.1474023 2083454.34315169 1468363.78038259
1326116.1692144 1314342.13126912 920802.61547049 780407.5175075
1678929.25641696 1173317.53334908 863938.9328098 831496.26628413
1552893.33508711 814232.28006267 628932.80758343 1230219.74082397
1009051.68059211 1134177.07317901 1759135.80092859 1185004.78325484
589995.18477429 1392467.64100483 1381608.25483992 1726383.2023419
1169019.22666496 1019912.31831202 1730374.72274501 1094660.75381921
1641553.67845519 1425844.82715513 1372526.00488651 957370.57751744
1758571.00815348 1215039.09536614 1228730.36130002 949454.36633531
1240479.54491375 1259976.01494439 1275292.19649843 1125707.61597875
1513693.61294972 1559271.12556507 798393.94186589 1546245.51538456
1278490.24324258 1048130.23839099 1258823.84340798 1647111.76109968
1110683.6841632 474642.39148037 1647293.82576375 1314423.54403662
1667425.25325174 1673875.47188459 1691912.07367635 1809235.34507848
1557560.5512079 872642.52777441 1383629.88700025 1588489.67118679
1394366.66112741 1126784.35366251 1453577.32336133 891270.72483827
929092.04974816 1124619.49617814 1189423.55209047 1093519.52228545
1352603.44998751 1104585.87337573 1041445.01140685 1840823.20915414
1269025.97772559 1195900.79478608 1982501.87427729 1172318.99196702
664063.71770282 1516831.57884726 1208883.11787161 1122128.21428225
1735594.69613153 865742.02321862 969966.3835537 1331559.38783137
909257.9004405 1330515.26483805 1267149.95713822 971617.92004141
1240075.55463063 1990811.03720136 1502950.35445567 1142677.03057216
1889344.61144088 1542683.11071993 1255495.76569687 1266858.02867607
1336567.39146564 250802.81939456 1128344.25997861 1251829.92396526
1365458.36530684 1731381.40176637 1048930.57357235 1054510.18009109
1426952.23586587 930090.24788653 866136.48384222 1648292.85950441
1183126.48975361 1342085.93169395 818757.06161575 1563303.58722225
1348611.35759588 1815034.40114857 1260025.43364773 1220361.24580727
648792.09698542 1331385.0939024 393964.58716725 1283083.6608295
1354368.39181222 1594675.47475904 1019367.99761248 1154895.92538237
1477596.50350931 1606153.12418652 1336855.88411859 1787639.82065234
1798694.76607084 945560.93733933 1240280.37820927 1131796.8979187
1506418.6409881 1172398.896648 1575031.41932382 1620022.75906772
328146.62358475 423405.29747082 808473.31516009 1808607.71767717
1161437.6335028 1290105.28066273 488495.93102175 1355523.83914854
1791747.74473713 530190.7597526 895829.94850127 1234872.22552441
1205813.61309886 1142869.05130465 1544709.4939468 1397728.97367436
809314.75267993 916515.5408125 1717159.66171621 1071915.80625235
1265594.03975673 1563516.24575786 1150899.12933388 1087806.80720891
1723353.35034214 1401272.11235719 1097379.04885339 1642119.10859682
1062758.32253009 1729910.73155036 1038760.9997124 1360748.10902365
1345562.75881699 1331901.19961839 1002669.41036228 1344417.49481131
1130066.69205505 1559820.77379468 1706430.10202814 2038885.49719396
833086.74048143 1045214.81139648 1134207.36502681 935557.862612
1372030.49915441 815056.03547679 1247496.29555842 1363128.23823989
1496484.74422308 2229545.19220177 777432.21374589 1191745.78488591
1096163.09011392 1272909.82942116 1625931.48842005 918993.98721316
1006465.36876058 1594755.7658865 846284.31587657 849305.27099749
570666.9766973 1801007.09806189 1485857.7010122 1686344.68445166
1231934.75994846 1356156.40905842 1097791.78972486 1193394.13367233
1126904.82603302 1084596.34251133 1044677.128424 2012287.09940269
749703.83822341 1125570.87522046 1353902.80662881 1582337.54477098
1132363.84344702 1127593.01594927 1558175.09336577 1470124.64995527
1170136.96370427 1057993.19774258 1727175.42480496 1099174.32885364
1878927.95333323 953041.98641305 220148.95728994 1469299.4012682
1351934.36007778 1965515.79787762 471073.53784835 1078285.07736102
1398197.71162202 1034751.6214149 792832.03083203 1446399.20930511
1103988.74382329 1691306.84800526 1107502.12092221 1078162.52791708
1708451.3561126 1134799.50112101 872219.41731097 871276.6337552
566610.74806608 1539564.17473228 1215068.56129604 1750435.57551414
780818.77892039 1005278.8908274 1699864.56225465 613683.19049426
1238072.22784607 1590149.31057521 1332467.65674073 1110842.05743917
1279563.18720233 894290.71453523 812546.8148645 1551251.04056016
1359009.91049348 1253121.43582776 1229760.03037545 1499246.99540704
919454.72722129 1331679.12723297 1089870.75329734 958885.84241772
1064647.10625584 941059.19510286 968260.24774319 1046073.30643464
1645969.84910694 1166194.65703568 1312898.64523883 1624075.1183887
1122680.60708451 1293518.53738823 1362243.66193653 1044593.86406878
1045450.3409926 1446228.97176106 1314918.61125719 1054569.81836383
1953570.35858134 1514052.36511509 1204362.0007214 1982821.65391243
680652.28599645 1723833.1028912 1461941.72132161 1413218.72506781
1074165.48822053 1048173.12975262 1205533.14320529 997083.01429422
1087634.17215582 799234.75302648 972455.40342001 801856.53463554
874552.70140583 1379123.09013505 1126134.74113496 1298148.81659145
1428161.98519949 1355123.02342546 1553045.32879594 1870468.89055136
941675.18754439 710776.75163677 1590857.45772608 1458290.81462446
666201.26424408 1740887.20683925 1311991.94997945 1798637.13769294
833944.9042026 1302260.14903338 1587128.52619347 1317721.78646457
1668052.02029736 936367.43448359 1700849.45729715 1165066.71552696
1555174.98797143 1203157.19294374 2182009.99760784 1050703.04898764
1085255.43002699 687418.2413657 1225471.31016553 1236416.18898843
1234673.47753594 930443.0490492 1501992.00715114 1273737.55032504
950041.90286064 1222288.62260244 1196391.39810298 1126494.323256
1430405.24832769 654124.8831567 895637.24071162 292231.65792553
1320679.35025207 805569.98785657 1233937.60765139 1104581.67567423
908260.05005094 1127651.17125846 1746143.30165373 550595.13591362
887562.56379595 1318241.03673407 1104224.94782251 1268614.59782285
773344.86318576 1279225.19247096 1409430.68554383 1438394.94609839
1242233.55899958 976371.07650901 961172.77960729 1405311.34334234
1231311.47373034 1416589.57175405 1039865.66760668 405050.76035601
1831567.93596 994168.26437392 1601760.17451136 1894119.37065231
838793.55050793 942753.23147991 2020611.40750524 892650.27370657
1103392.89712649 1856735.83501372 1374941.34714029 1347030.9418317
839567.56053583 1868960.81804402 1046950.66016825 837784.75799152
1243870.52458918 945414.8784892 1003374.52464082 1290578.27919801
1173331.32430923 1522296.87016011 1104182.96400372 1284736.34065318
666922.2209623 673548.38060283 839842.87494546 1485091.87949437
1758926.1001963 616983.40087472 1267547.14699049 899323.40602058
1158839.68496783 754436.13286385 1150948.88850265 1255846.0700254
771868.76930345 1323417.05382824 929827.54051279 1067842.89483073
1110264.9618046 1540579.01469861 806070.2039711 1375018.45965384
1583012.8637186 1215804.72853409 1846871.22490026 1719674.14920754
1072371.6108295 1261854.33505624 1801461.10335271 1289458.68342156
1371299.08117235 983760.56441569 1324124.02939088 1051323.27262374
1007052.9443188 1401321.32709743 1571085.17286577 1229001.87119959
1283186.61191567 1288554.56408168 1415412.18859016 1103447.66939766
1650111.15596409 1351906.62870447 806922.81052761 1101879.75531369
1087454.15325061 1407522.12276368 1458792.37938237 1472979.40100164
1430788.15060835 1233623.36217147 1870533.17388317 1257158.33660787
1600913.12822158 1356762.37639189 1319817.63503686 1236051.9108198
1268181.38595477 1129570.75163033 1705669.67477113 1411102.91700132
2273540.41800102 1207547.00425412 899781.3847036 1254140.99658748
1542438.22564716 657936.46719116 1385268.91742449 878970.16758734
1109196.43128029 1353391.17019487 1696115.98345093 1413282.72684313
1827087.79734247 1120215.62692099 1356976.31422317 1223780.10870815
1248092.82209771 1769843.13529577 949225.39612295 1477952.65052356
1255282.59862256 426003.13583316 707530.77134158 1696903.01183506
1163050.51049259 1323411.5033268 2075338.18777907 1574543.41531418
1817164.81632782 1027880.42644512 984572.34397046 1347495.61247839
1405652.70637065 1378100.57654898 1725502.2933008 1380099.65837807
1577843.39344022 1624768.07507459 620250.96994802 678847.62293504
1255463.63367927 1493178.26766321 1251118.57109733 1531225.73325
1038742.17013484 158813.64470869 670803.74477208 1358866.25754787
1116231.80776672 825567.42192957 1205988.87074548 1163767.67010631
1795770.41249053 1037902.14643255 1066195.46094948 1082294.65493931
1435062.76289096 1454466.64232999 1330792.13240183 1690582.50968939
1069251.9487768 808110.53863472 625553.6464463 919490.05366638
1353662.2546001 1184444.42780225 1539245.25567221 1208513.48652763
837956.30959035 1659341.88709898 1392103.22796819 1589783.52479679
1262963.45793841 1511554.88048253 883619.90417901 1022564.2478265
2053151.68156221 1324454.22123298 1339389.73837403 916369.74594413
1080360.83870116 1124354.20250161 1225725.51762075 1036709.01383128
1234858.0860976 1055632.34784629 1517840.47571422 1327306.74681115
1172875.46526553 663510.22702194 994880.08825416 659636.17716454
1215833.3865498 1289298.54249165 1049066.68537325 1002058.65544564
1479008.16442341 1133499.87344621 1343292.37992994 1240281.28609788
1875671.59202051 1384525.66937356 900349.97821838 1491477.28606211
291738.12188895 1146285.9434131 1367218.08956031 1289910.89226899
1291184.80884029 1824408.57177525 1491241.2227773 1389476.45607064
1321293.64559164 1284967.15702986 846503.99338371 1311564.60147658
1451747.22228778 1884449.22275646 1289541.93048528 794205.10442291
1192680.65890835 1126098.68560079 1509907.60069687 1913607.45329345
829309.12969399 595859.28942419 1582813.3055729 1035614.81747863
1456522.8591344 925110.32853942 1095178.12785018 1245801.01775937
1464082.99859734 1554146.7720129 965107.42154312 1356373.22425334
1673565.88692989 1158338.71531108 1281278.86098632 787926.23885863
1408326.67504604 1269972.82557244 440821.15599144 1191806.26503558
856260.95454576 1026274.59180668 915461.63191209 1813981.92931032
1260397.95598962 1238979.10170183 1387410.70388008 804543.00233416
1184273.71677171 895553.53733314 666753.31379016 494113.56401944
1433820.74136242 920519.49342551 1552699.63507721 1314958.29825851
1408137.11007595 1105205.03868602 1225374.9668051 1555967.60398297
764356.87177392 1209380.67918476 1307446.07617411 1156888.49949617
1477617.77388599 1570107.70957062 888604.61517341 1357192.46882878
1122559.1071572 1611585.4588343 1055610.51785009 1553877.5957549
1094835.14060777 925610.91139446 1443072.82447055 887534.1374447
456214.10636656 1667261.18226071 1271904.57635987 1182679.40285231
1649171.89032533 1423009.73879234 1484679.82766679 1033297.25906527
1292529.61451445 1145394.95691419 978455.62438492 1249136.06087141
1127225.37341876 892653.77412716 1511054.90056614 873016.64861266
776802.43645933 1039353.56986007 1315157.46162109 1584289.80977678
987849.3901897 1368365.93287136 1100557.38102402 1365918.94448344
935645.93243935 324562.71318829 882741.4389428 1276731.80519713
1198249.83684015 1049018.28659815 636396.7037124 1449632.62265719
1383995.417274 1298088.72377639 1540539.70367801 1493896.99889277
2343746.35475218 1872235.70486221 1439963.7293974 764711.28781408
800048.08135589 1913553.84633185 788172.68793124 1569240.63551064
1490513.58848105 1140794.46978976 1258627.64263355 711243.70457631
1250422.00060581 1164795.5837308 1719556.05365748 1144266.71457958
1494354.61963915 1483904.82826235 1384296.65337772 2120401.56572417
1436436.14421377 1360879.56964684 948664.59412427 1531287.09616398
891575.11288498 1323714.31616798 743700.71602049 1226590.09491137
990596.20736163 1233992.23613085 1418498.89087371 1538321.56247525
496671.07127634 1441826.36086485 1807086.36903315 1288631.96182057
1067396.35435427 1234051.48903035 1510931.44172461 1238596.80367902
1079587.86267727 1096446.24680233 1340780.37376665 1728349.44751938
1182885.47597413 1396880.83572716 848540.01935768 1118181.60414617
1450084.64438066 2095131.06695887 1432554.8369338 1097488.60717876
1543895.07644596 589701.16866297 1632891.15370816 1462326.34718035
1251619.86424647 1215142.06779053 887591.37509515 1012091.21646187
1528992.42455722 1224454.45215524 1657636.49616451 1521618.14196433
1395801.01427134 1559056.91331875 1474320.2159518 1266083.75708837
598008.41675619 775371.04758185 1626814.6124692 1184704.01403565
1378438.85088416 1999851.71462622 519552.57086123 939469.86749699
1730275.64303092 787500.26420787 1266092.51639942 1334783.79526669
1311501.65110258 790924.30378168 1701593.43687928 1031290.33368498
1384964.63414445 1881154.94357247 1135281.19135162 1155858.13118106
821938.51015299 1392569.03068382 1828630.6890108 1499599.51634769
1768913.65916671 2140238.41934353 1312065.46959489 1297778.51810689
954090.00438522 1164766.05704707 1762265.19883864 1272190.22341176
1337046.93436166 1734432.16375048 1241997.26902784 1545171.11180707
1410803.40672303 842583.16037094 1410431.15875628 843468.41686778
963938.51049702 1359381.61691094 1086200.9207718 1724349.54285902
1488902.61977152 1435137.84302696 2032773.75166519 1550293.84023723
1117672.92731721 1228981.23802782 1024338.06123588 1152686.83818179
1016242.46686364 1375666.28141413 1050507.27599655 1181790.30430949
1525713.82292139 1681606.23746156 1205200.14071651 1473170.42751321
1290698.64726292 1157017.91864223 1637827.88146071 591770.21795497
1900211.94766033 1461556.14927682 1338718.53329421 1365231.39271031
966650.94972922 1677602.58059847 1401419.04822992 2018052.80955535
1183950.82427373 1450992.61747403 1177265.33458142 843749.10312845
1497536.13019635 1529073.71346475 558964.87508147 1261583.2192759
1753273.50402693 1500926.03391635 1059402.18036569 1709352.5707534
1112889.9392433 1247534.54359931 853834.333056 706144.92549257
1070761.99800867 1659159.91706308 1062693.4703681 1073545.82718401
1036990.30062324 1052078.86312731 1568784.39882065 1309154.63561277
858450.71935711 988956.07442349 1396520.63086133 1769711.99813365
1283298.07540821 1515402.04421687 1256935.59508284 1320333.17632623
1505221.09859151 1110347.82733454 771327.59692895 1189663.34806261
1128591.58671394 1533502.4634133 2187616.08323863 1177106.83506023
1490349.58869886 1410251.5440795 1461916.30494554 1548793.63000759
2006242.92462349 1312408.85291948 1581878.60451192 631870.52706524
2015104.50190244 1056903.78936517 1547405.20803519 875505.84365161
1826751.87241242 1174567.82091767 844395.35409811 1682709.20725988
1275129.63095662 1526887.72765502 1000089.93827973 1529941.81041016
520249.23833003 1509899.51986588 1245221.05841925 1415065.50463792
1664533.90291694 1299536.2446968 1475156.35270561 1587824.19159122
1087189.99440689 1242310.46082559 932496.32274209 1457832.98702953
1327013.30958709 1365624.93545962 1065213.12237315 1097280.88986157]
Bước 7: Đánh giá chất lượng mô hình máy học
Trước tiên ta xem sự tương quan hệ số coefficients và interpret:
# print the intercept
print(lm.intercept_)
coeff_df = pd.DataFrame(lm.coef_, X.columns, columns=['Coefficient'])
print(coeff_df)
Thực thi lệnh trên ta có kết quả:
-2640441.3997814017
Coefficient
Avg. Area Income 21.566696
Avg. Area House Age 165453.042478
Avg. Area Number of Rooms 120499.839093
Avg. Area Number of Bedrooms 1999.785336
Area Population 15.340604
Coeffient cho ta thấy:
- Giả sử các thuộc tính khác không đổi, nếu Area Income tăng D đơn vị thì giá của căn nhà sẽ được bổ sung thêm D*21.5666696
- Tương tự cho các thuộc tính khác. Như vậy dựa vào Cofficient ta biết được mức độ ảnh hưởng của các biến độc lập lên biến phụ thuộc. Ví dụ trong trường hợp này thì rõ ràng là tuổi thọ của căn nhà có tầm ảnh hưởng về giá của căn nhà lớn nhất, và dân số đóng vai trò thấp nhất về sự ảnh hưởng giá.
Ta có một số cách để đánh giá mô hình dự báo chạy bằng Hồi quy như sau:
Mean Absolute Error (MAE): MAE là một phương pháp đo lường sự khác biệt (độ chênh lệch giá trị) giữa hai biến liên tục. Giả sử rằng X và Y là hai biến liên tục thể hiện kết quả dự đoán của mô hình và kết quả thực tế, công thức:
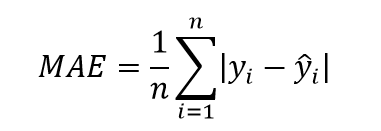
Mean Squared Error (MSE): là giá trị trung bình của bình phương sai số (Hàm mất mát), là sự khác biệt giữa các giá trị được mô hình dự đoán và gía trị thực. MSE cũng được gọi là một hàm rủi ro, tương ứng với giá trị kỳ vọng của sự mất mát sai số bình phương hoặc mất mát bậc hai chỉ số này phổ biến hơn chỉ số MAE bên trên, công thức:
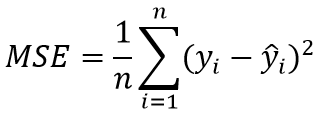
Root Mean Squared Error (RMSE): là căn bậc hai của giá trị trung bình của các sai số bình phương (MSE). Thông thường, ta thường dùng chỉ số này để xác định giá trị chênh lệch trung bình giữa giá dự đoán và giá trị test ban đầu, công thức:
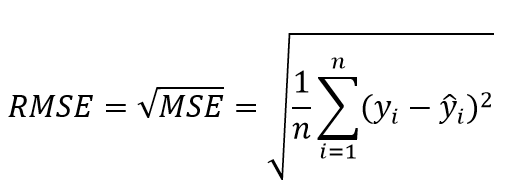
print('MAE:', metrics.mean_absolute_error(y_test, predictions))
print('MSE:', metrics.mean_squared_error(y_test, predictions))
print('RMSE:', np.sqrt(metrics.mean_squared_error(y_test, predictions)))
Thực thi lệnh trên ta có kết quả:
MAE: 81305.23300085647
MSE: 10100187858.864885
RMSE: 100499.69083964829
Tùy vào lựa chọn tỉ lệ train mà các giá trị đo lường này có thể khác nhau.
Bước 8: Kết xuất mô hình máy học ra ổ cứng (file zip) để tái sử dụng
Khi mô hình được đánh giá là chạy tốt, ta nên lưu lại mô hình để các lần sau sử dụng chỉ cần nạp lại mô hình thay vì phải train lại (công đoạn train thường là tiêu tốn nhiều thời gian và nguồn lực).
Lưu mô hình, mô hình ở trên được đặt tên biến là lm, ta cần lưu mô hình lm này xuống ổ cứng để sử dụng cho lần sau, sử dụng thư viện pickle:
modelname = "housingmodel.zip"
pickle.dump(lm, open(modelname, 'wb'))
Thư thi lệnh trên ta sẽ có mô hình được lưu xuống ổ cứng với tên “housingmodel.zip”:
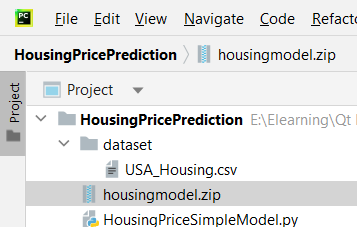
Source code đầy đủ của “HousingPriceSimpleModel.py”:
# 01.Library for dataset processing
import pandas as pd
import numpy as np
# 02. Library for train model
from sklearn.model_selection import train_test_split
from sklearn.linear_model import LinearRegression
# 03. Library for evaluation model
from sklearn import metrics
# 04. Library for saving model
import pickle
#use pandas to read CSV dataset
df = pd.read_csv('dataset/USA_Housing.csv')
#call functions about get dataset information:
print(df.head())
print(df.info())
print(df.describe())
print(df.columns)
#set X matrix
#df.columns[:5] meaning:
#['Avg. Area Income', 'Avg. Area House Age', 'Avg. Area Number of Rooms',
# 'Avg. Area Number of Bedrooms', 'Area Population']
X = df[df.columns[:5]]
y = df['Price']
# Printing for observation:
print(X)
print(y)
X_train, X_test, y_train, y_test = train_test_split(X, y, test_size=0.2, random_state=101)
lm = LinearRegression()
lm.fit(X_train, y_train)
print("Input 1:")
print([X_test.iloc[0]])
pre1 = lm.predict([X_test.iloc[0]])
print("Housing Price prediction 1 =", pre1)
print("Input 2:")
input2=[66774.995817, 5.717143, 7.795215, 4.320000, 36788.980327]
print(input2)
pre2 = lm.predict([input2])
print("Housing Price prediction 2 =", pre2)
print("Input 3:")
input3=[21.566696, 165453.0425, 120499.8391, 1999.785336, 15.340604]
print(input3)
pre3 = lm.predict([input3])
print("Housing Price prediction 3 =", pre3)
predictions = lm.predict(X_test)
print("Full Housing Price Predictions:")
print(predictions)
# print the intercept
print(lm.intercept_)
coeff_df = pd.DataFrame(lm.coef_, X.columns, columns=['Coefficient'])
print(coeff_df)
print('MAE:', metrics.mean_absolute_error(y_test, predictions))
print('MSE:', metrics.mean_squared_error(y_test, predictions))
print('RMSE:', np.sqrt(metrics.mean_squared_error(y_test, predictions)))
modelname = "housingmodel.zip"
pickle.dump(lm, open(modelname, 'wb'))
Bước 9: Sử dụng/Tái sử dụng mô hình máy học, tạo thêm 1 file mã lệnh Python “UseHousingPriceSimpleModel.py”

Bổ sung mã lệnh cho “UseHousingPriceSimpleModel.py”
import pandas as pd
import pickle
from pandas import Index
modelname="housingmodel.zip"
# Load Trained Machine Learning Model
trainedmodel=pickle.load(open(modelname, 'rb'))
features=Index(['Avg. Area Income', 'Avg. Area House Age', 'Avg. Area Number of Rooms',
'Avg. Area Number of Bedrooms', 'Area Population'],
dtype='object')
# Check coeff again if you want (no need)
coeff_df = pd.DataFrame(trainedmodel.coef_,features,columns=['Coefficient'])
print(coeff_df)
print("Input 1:")
input1=[66774.995817,5.717143,7.795215,4.320000,36788.980327]
print(input1)
prediction1=trainedmodel.predict([input1])
print("Housing Price Prediction 1=",prediction1)
print("-"*50)
input2=[[66774.995817,5.717143,7.795215,4.320000,36788.980327],
[80527.47208,8.093512681,5.0427468,4.1,47224.35984]]
print(input2)
prediction2=trainedmodel.predict(input2)
print("Housing Price Prediction 2=",prediction2)
Mã lệnh ở trên sẽ tải mô hình máy học đã được lưu trước đó “housingmodel.zip” và tiến hành tái sử dụng để predict giá nhà, chạy mã lệnh trên ta có kết quả:
Coefficient
Avg. Area Income 21.566696
Avg. Area House Age 165453.042478
Avg. Area Number of Rooms 120499.839093
Avg. Area Number of Bedrooms 1999.785336
Area Population 15.340604
Input 1:
[66774.995817, 5.717143, 7.795215, 4.32, 36788.980327]
Housing Price Prediction 1= [1257919.71744578]
--------------------------------------------------
[[66774.995817, 5.717143, 7.795215, 4.32, 36788.980327], [80527.47208, 8.093512681, 5.0427468, 4.1, 47224.35984]]
Housing Price Prediction 2= [1257919.71744578 1775665.87664153]
Như vậy tới đây Tui đã hướng dẫn xong các bạn cách thứ sử dụng thư viện Hồi quy tuyến tính để train một mô hình máy học đơn giản để dự báo giá nhà, các bạn biết cách/ôn tập pandas để đọc và tiền xử lý dữ liệu, biết cách dùng train_test_split, LinearRegression để train mô hình. Biết cách dùng metrics để đánh giá chất lượng mô hình cũng như dùng pickle để lưu mô hình và phục hồi lại mô hình để tái sử dụng trong tương lai.
Source code đầy đủ của bài này bạn tải ở đây:
https://www.mediafire.com/file/z0yp8561ztuiaz5/HousingPricePrediction.rar/file
Bài học sau Tui sẽ hướng dẫn các bạn thiết kế màn hình tương tác người dùng để train mô hình cũng như sử dụng mô hình máy học trên giao diện. Các bạn chú ý theo dõi
Chúc các bạn thành công